IoTSim-Osmosis
Osmotic computing paradigm sets out the principles and algorithms for simplifying the deployment of Internet of Things (IoT) applications in integrated edge-cloud environments. Osmotic Computing focuses on strategies and mechanisms to extend the IoT capabilities by defining, designing, and implementing a modern computing model (IoT, edge, cloud, and SD-WAN).
IoTSim-Osmosis is a simulation framework that supports the testing and validation of osmotic computing applications. In particular, it enables a unified modelling and simulation of complex IoT applications over heterogeneous edge-cloud SDN-aware environments. IoTSim-Osmosis is capable of capturing the key functions, characteristics, and behaviors of osmotic paradigm. A wide range of osmosis applications can be simulated and evaluated in IoTSim-Osmosis. To handle the complexity and diversity of osmotic applications, IoTSim-Osmosis provides an abstract mechanism called Microelements (MELs), which encapsulates services, resources and data. In particular, any IoT applications can be represented using a graph of MELs as shown in the figure below.
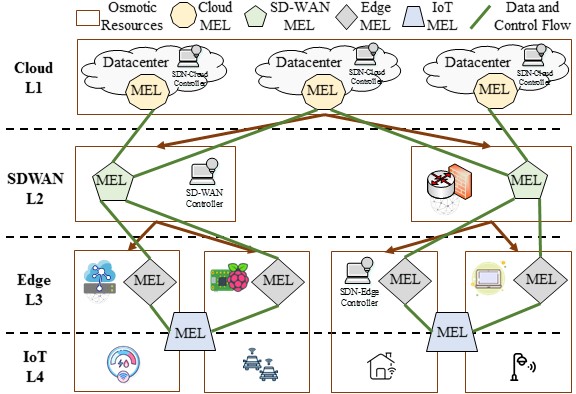
Unique Features
IoTSim-Osmosis is developed to allow such hybrid infrastructures to be simulated. The dynamic management and performance metrics of IoT-oriented services across edge and cloud datacentres that communicate via SDWAN are easily achieved. In particular, IoTSim-Osmosis is capable of modeling and simulating:
- Osmotic applications running between edge and cloud
- The behaviors and features of osmotic applications running in dynamic SDN and SD-WAN networks
- Dynamic routing mechanisms based on graph theory to enable any type of network topology to be seamlessly simulated
- Several policies for SDN, SD-WAN, and MEL, VM for multilevel optimization
Download
Find the following links to download the simulator and user manual to start work on it.
- IoTSim-Osmosis __ Code [LINK]
- IoTSim-Osmosis __ User manual [LINK]
- IoTSim-Osmosis __ Introduction Video [Coming Soon!]
Support email: Khaled Alwasel (kalwasel@gmail.com)
Scientific References
Khaled Alwasel, Devki Nandan Jha, Fawzy Habeeb, Umit Demirbaga, Omer Rana,Thar Baker, Scharam Dustdar, Massimo Villari, Philip James, Rajiv Ranjan, “oTSim-Osmosis: A Framework for Modelling & Simulating IoT Applications Over an Edge-Cloud Continuum,” Journal of Systems Architecture. (to be online soon)
BigDataSDNSim
BigDataSDNSim is a discrete-event simulation tool designed to enable the modeling and simulation of Big Data Management Systems (BDMS such as Apache Hadoop YARN), MapReduce programming model, and Software Defined Network (SDN)-enabled networks within cloud computing environments. It is the first tool that models and simulates the three merging technologies (MapReduce-BDMS, Stream-BDMS, SDN, cloud) in a single simulator. The simulator is capable of capturing the key functions, characteristics, and behaviors of the SDN-enabled MapReduce computing environment. It is also capable of modeling the functionalities of MapReduce applications in line with mimicking diverse SDN capabilities and interactions with BDMS systems in a seamless manner. The conceptual architecture of the simulator is shown below.
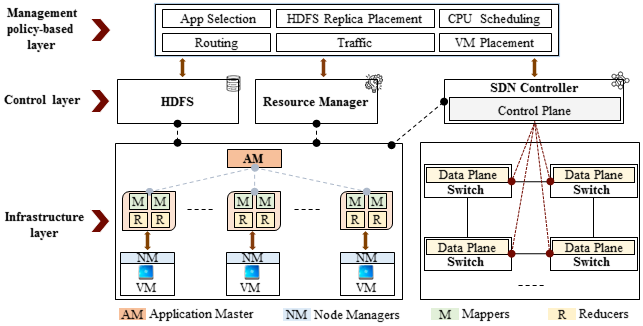
Unique Features
BigDataSDNSim differs from other simulation tools in supporting a holistic simulation framework that simulates MapReduce applications, BDMS, and SDN-related networks in cloud-based environments. In particular, BigDataSDNSim is capable of modeling and simulating:
- A generic big data approach for executing different big data programming models (e.g. MapReduce, Stream) simultaneously;
- MapReduce applications within big data cluster management (BDMS), which is one of the key prominent framework for running different big data models;
- The behaviors and features of SDN dynamic networks coupled with the coordination and interaction with MapReduce applications within cloud environments;
- Dynamic routing mechanisms based on graph theory to enable any type of network topology to be seamlessly simulated;
- Modeling several policies for SDN, MapReduce, and VM within cloud data centers for multilevel optimization;
- Variety of cloud data center nodes combined with SDN controllers to satisfy the requirements of big data applications
Download
Find the following links to download the simulator and user manual to start work on it.
- BigDataSDNSim __ Code [LINK]
- BigDataSDNSim __ User manual [LINK]
- BigDataSDNSim __ Introduction Video [Coming Soon!]
Support email: Khaled Alwasel (kalwasel@gmail.com)
Scientific References
Khaled Alwasel, Rodrigo N. Calheiros, Saurabh Garg, Rajkumar Buyya, and Rajiv Ranjan, “BigDataSDNSim: A Simulator for Analyzing Big Data Applications in Software-Defined Cloud Data Centers“, https://arxiv.org/pdf/1910.04517.pdf.
IoTSim-Edge
IoTSim-Edge simulator provides the following features to simulate the IoT and edge environment.
- Variety of IoT devices need to be combined with edge IoT gateways to satisfy the requirements of an application
- Modeling networking graph between diverse type of IoT and edge computing device in an abstract manner can be very challenging
- Modeling data and control flow dependencies between IoT and Edge layers to support diverse data analysis work-flow structure is non-trivial
- Capacity planning across edge computing layer is challenging as it depends on various configuration parameters including data volume, data velocity, upstream/downstream network bandwidth, to name a few
- The communication between IoT and edge devices is very different from cloud datacenter communication, which is generally based on wired and/or wireless protocol.
- The energy model of sensor nodes
- Modeling the mobility pattern of devices dynamicity of IoT environment
IoTSim-Edge simulator also models the distribution and processing of streaming data generated by IoT devices in the Edge computing environment.
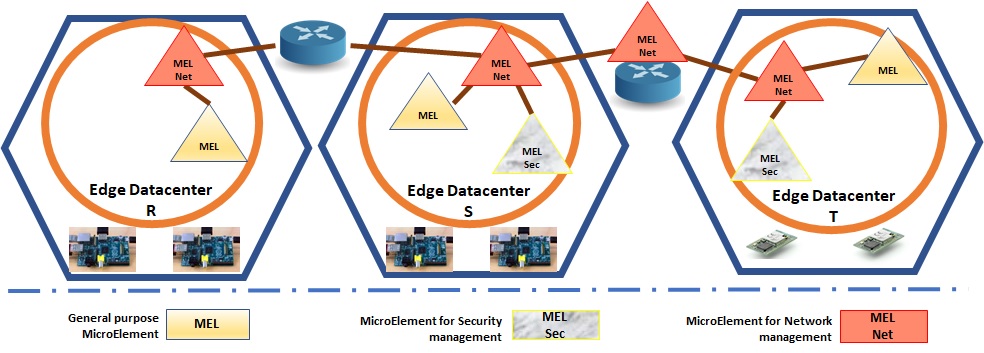
Above figure describes The IoTSim-Edge sytstem model. To better describe the management of computing, the computing environments are divided into two main layer 1 (L1), edge systems and microdata centers; and layer 2 (L2), IoT devices. The IoTSim-Edge also supports of modelling of data analysis tasks as MicroElements (MELs) – a fundamentaly new graph-based abstraction to model and program IoT applications.
Unique Features
- New IoT application graph modeling abstraction that allows practitioners to define the data analytic operations and their mapping to different parts of the infrastructure (e.g. IoT and edge).
- The abstraction that supports modeling of heterogeneous IoT protocols along with their energy consumption profile.
- It allows practitioners to define the configuration of edge and IoT devices along with the specific protocols they support for networking.
- The abstraction that supports modeling of mobile IoT devices.
- It also captures the effect of handoff caused by the movement of IoT devices. This supports a cooperative edge-to-edge communication that allows the transfer of the processed data of the respective IoT device by one edge via another edge.
Download
Find the following links to download the simulator and user manual to start work on it.
- IoTSim-Edge __ Code [LINK]
- IoTSim-Edge __ User manual [LINK]
- IoTSim-Edge __ Introduction Video [LINK]
Support email: Khalid Alwasel (kalwasel@gmail.com), Deepak Puthal (dputhal88@gmail.com) and Devki Nandan Jha (i.dnjha@gmail.com)
Scientific References
- X. Zeng, S. K. Garg, P. Strazdins, P. Jayaraman, D. Georgakopoulos and R. Ranjan, “IOTSim: a Simulator for Analysing IoT Applications,” Journal of System Architecture, Elsevier. [ISI Impact Factor: 0.44] (Accepted July 2016)
- G. Kecskemeti, G. Casale, D. N. Jha, J. Lyon, R. Ranjan, “Modelling and Simulation Challenges in the Internet of Things,” IEEE Cloud Computing, IEEE Computer Society. (To Appear January 2017, Reviewed by Editorial Board) [ISI Impact Factor: 4.39]
- D. N. Jha, K. Alwasel, A. Alshoshan, X. Huang, R. K. Naha, S. K. Battula, S. Garg, D. Puthal, P. James, A. Y. Zomaya, S. Dustdar, and R. Ranjan, “IoTSim-Edge: A Simulation Framework for Modeling the Behaviour of IoT and Edge Computing Environments“, Software: Practice and Experience, 2019. (Minor Revision May 2019)
IoTSim-Stream
IoTSim-Stream is an IoT Simulator for Stream processing on the big data that offers an environment to model complex stream graph applications in Multicloud environment, where the large-scale simulation-based studies can be conducted to evaluate and analyze these applications. It leverages the features of CloudSim and integrating real-time processing model with workflow scheduling and execution to execute the modeled stream graph application in Multicloud environment.
IoTSim-Stream supports the modelling of different patterns/structures of stream workflow applications, which are linear (a multi-stage application where each stage processes input stream generated by the previous stage and produces the output stream to the following stage), branching (an application with limited precedence constraints that splits data stream to perform different parallel processing and then combining the results for further analysing) and hybrid (a mix of linear and branching patterns).
Above figure shows simple instance of large-scale datastream-processing service that an be simulated and modelled using IoTSim-Stream. The example service consists of Apache Kafka (data ingestion layer), Apache Storm (data analytics layer), and Apache Cassandra Systems (data storage layer).
Unique Features
- Support modeling data incentive IoT-based applications using stream processing model (aka stream graph applications).
- Support modeling Multicloud environment as an execution environment for stream graph application.
- Support user-defined resource provisioning and scheduling policies.
Download
Find the following links to download the simulator and user manual to start work on it.
- IoTSim-Stream __ Code [LINK]
- IoTSim-Stream __ User manual [LINK]
- IoTSim-Stream __ Introduction Video [LINK]
Support email: Mutaz Barkia (mutaz.barika@utas.edu.au) and Khalid Alwasel (kalwasel@gmail.com)
Scientific References
- M. Barika, S. Garg, A. Chan, R. N. Calheiros and R. Ranjan, “IoTSim-Stream: Modeling Stream Graph Application in Cloud Simulation,” Future Generation Computer Systems Journal, Elsevier Press. [ERA A Journal, ISI impact factor: 3.99] (accepted April 2019, in press)
- X. Zeng, S. K. Garg, P. Strazdins, P. Jayaraman, D. Georgakopoulos and R. Ranjan, “IOTSim: a Simulator for Analysing IoT Applications,” Journal of System Architecture, Elsevier. [ISI Impact Factor: 0.44] (Accepted July 2016)